More Guns Less Crime, John Jr [free novels TXT] 📗
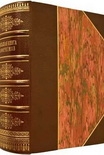
- Author: John Jr
Book online «More Guns Less Crime, John Jr [free novels TXT] 📗». Author John Jr
Several other general concerns may be anticipated in setting up the regression specification. What happens if concealed-handgun laws just happen to be adopted at the same time that there is a downward national trend in crime rates? The solution is to use separate variables for the different years in the sample: one variable equals 1 for all observations during 1978 and zero for all other times, another equals 1 for all observations during 1979 and zero otherwise, and so on. These "year-dummy" variables thus capture the change in crime from one year to another that can only be attributed to time itself. Thus if the murder rate declines nationally from 1991 to 1992, the year-dummy variables will measure the average decline in murder rates between those two years and allow us to
ask if there was an additional drop, even after accounting for this national decline, in states that adopted nondiscretionary concealed-handgun laws.
A similar set of "dummy" variables is used for each county in the United States, and they measure deviations in the average crime rate across counties. Thus we avoid the possibility that our findings may show that nondiscretionary concealed-handgun laws appear to reduce crime rates simply because the counties with these laws happened to have low crime rates to begin with. Instead, our findings should show whether there is an additional drop in crime rates after the adoption of these laws.
The only way to properly account for these year and county effects, as well as the influences on crime from factors like arrest rates, poverty, and demographic changes, is to use a multiple-regression framework that allows us to directly control for these influences.
Unless we specifically state otherwise, the regressions reported in the tables attempt to explain the natural logarithms of the crime rates for the different categories of crime. Converting into "logs" is a conventional method of rescaling a variable so that a given absolute numerical change represents a given percentage change. (The familiar Richter scale for measuring earthquakes is an example of a base-10 logarithmic scale, where a tremor that registers 8 on the scale is ten times as powerful as one that registers 7, and one that registers 7 is ten times as powerful as one that registers 6.) The reason for using logarithms of the endogenous variable rather than their simple values is twofold. First, using logs avoids giving undue importance to a few, very large, "outlying" observations. Second, the regression coefficient can easily be interpreted as the percent change in the endogenous variable for every one-point change in the particular explanatory variable examined.
Finally, there is the issue of statistical significance. When we estimate coefficients in a regression, they take on some value, positive or negative. Even if we were to take two completely unrelated variables—say, sun-spot activity and the number of gun permits—a regression would almost certainly yield a coefficient estimate other than zero. However, we cannot conclude that any positive or negative regression coefficient really implies a true relationship between the variables. We must have some measure of how certain the coefficient estimate is. The size of the coefficient does not really help here—even a large coefficient could have been generated by chance.
This is where statistical significance enters in. The measure of statistical significance is the conventional way of reporting how certain we can be that the impact is different from zero. If we say that the reported number is "positive and statistically significant at the 5 percent level," we mean that there is only a 5 percent chance that the coefficient happened to
APPENDIX ONE/249
take on a positive value when the true relationship in fact was zero or negative. 2 To say that a number is statistically significant at the 1 percent level represents even greater certainty. The convention among many social scientists is usually not to affirm conclusions unless the level of significance reaches 10 percent or lower; thus, someone who says that a result is "not significant" most likely means that the level of significance failed to be as low as 10 percent.
These simple conventions are, however, fairly arbitrary, and it would be wrong to think that we learn nothing from a value that is significant at "only" the 11 percent level, while attaching a great deal of weight to one that is significant at the 10 percent level. The true connection between the significance level and what we learn involves a much more continuous relationship. We are more certain of a result when it is significant at the 10 percent level rather than at the 15 percent level, and we are more certain of a result at the 1 percent level than at the 5 percent level.
Appendix Two
Explanations of Frequently Used Terms
Arrest rate: The number of arrests per crime.
Crime rate: The number of crimes per 100,000 people.
Cross-sectional data: Data that provide information across geographic areas (cities, counties, or states) within a single period of time.
Discretionary concealed-handgun law: Also known as a "may-issue" law; the term discretionary means that whether a person
Comments (0)